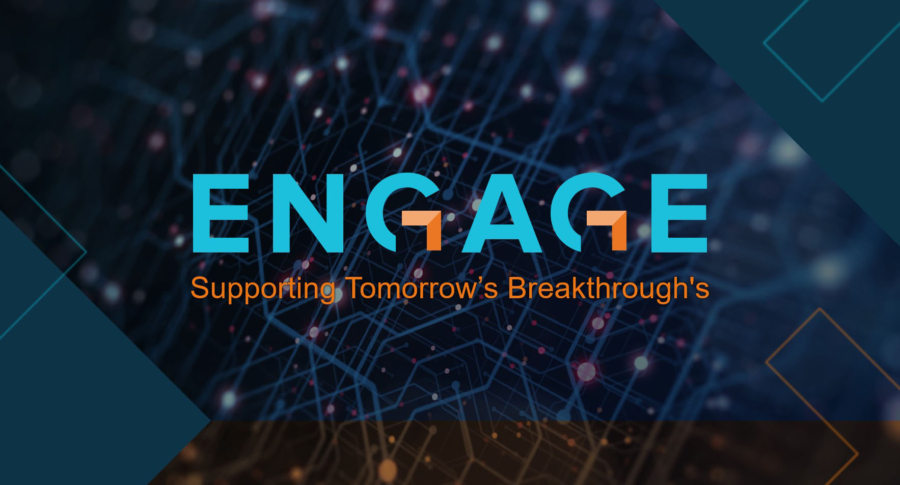
AI/ML in Clinical Data Analytics, Innovation Series Episode 1 Recap
There’s no question that the buzzword of the moment in life sciences is artificial intelligence, as many clinical and operational teams are working toward leveraging automation technology to increase efficiency and achieve faster deliverability in trials. However, for companies to stay agile and future-ready we must look deeper through the AI hype and think critically about its benefits and ways to overcome potential roadblocks along the way.
In Episode 1 of the eClinical Innovation Podcast Series with guest, Sandy Ng, VP of Data Sciences at eClinical Solutions, we examined the skepticism around integrating AI/ML into existing data strategies and shared real use cases of how our internal data management team has applied AI functionality with elluminate® Clinical Data Cloud users.
The Innovation Podcast Series was designed to bring you the latest on AI/ML and other leading innovations that are impacting clinical development. Featuring industry experts and hosted by Venu Mallarapu, VP of Global Strategy & Operations at eClinical, the podcast highlights R&D efficiency in data analytics, trial optimization, and more. Subscribe to the series to ensure you never miss an episode.
Listen
A Summary of Podcast Series Q&A with Sandy Ng
Venu Mallarapu: Briefly, could you share a little about your background and your role as VP of Data Sciences at eClinical Solutions?
Sandy Ng: I have a doctorate in engineering and started off my career as a biomedical engineer, working on algorithm development for sensors in medical devices, transitioning into the healthcare and clinical trials space. For example, building prognostic models for diseases and, for clinical trials, using sensors and sensor data for DCTs.
Here at eClinical Solutions, my focus is on driving product innovation using AI/ML-enabled techniques, to identify opportunities to solve the pain points of our clients and make the clinical trial process easier and faster. I lead a team of data scientists in AI/ML model development, validating hypotheses, testing new features, and measuring performance to ensure that our products are continually evolving and that they address client needs.
Venu: Looking at today’s clinical trial landscape, in your opinion why is AI/ML relevant, and why should it be applied in Clinical Data Analytics in the first place?
Sandy: I would liken AI/ML techniques to a diverse set of tools in a toolbox, not the only tools in the toolbox, but they offer us a wider range of options to address the complex challenges that our clinical development clients face. In general, AI/ML is good for automating tasks that are repetitive and manual like data checks, and for tasks that are hard for humans, like predicting outcomes. Some examples of problems that AI/ML techniques are suited for are uncovering patterns in the data that might be challenging for humans to identify, forecasting future trends, and predicting outcomes based on historical data, anomaly detection to identify unusual patterns or outliers in data, and NLP to extract relevant information from unstructured text.
Venu: What are some of the problems/use cases your team has solved for, as part of the elluminate Clinical Data Cloud?
Sandy: I’ll share 3 examples of how we’ve already implemented AI/ML in elluminate which focus on data review automation to improve data quality and the speed of review. The first is the detection of abnormal changes in lab values and vital signs for a given patient. A large increase in lab value, say total bilirubin count, between visits, for example. These may be indicative of data entry errors or safety signals. The second is the detection of concomitant medication (CM) records that may have incorrect entries for dose unit or route. That is, the unit or route may be inconsistent with the rest of the entries in the CM record. We flag those and we also suggest what the matching learning model predicts is the correct unit or route. The third is the detection of whether a record entered as an adverse event (AE)or medical history (MH) is truly an AE or MH, and not a procedure instead. This is a check to identify records that may be entered in the incorrect form or domain or may be incorrectly coded.
Venu: What kind of Machine Learning models and approaches have you used in solving these problems?
Sandy: We use both supervised and unsupervised learning, depending on the use case and the available data. We train on historical data when appropriate. The techniques we’ve implemented so far include anomaly detectors, gradient boosted machines, and natural language processing.
Venu: Can you share some of the challenges that your team has encountered, and what steps you took to overcome them?
Sandy: The first challenge is truly understanding what the most valuable use cases are in terms of what we should work on and what would help our clients most. Luckily, we have internal teams like our Technical Services and Biometrics Services teams where we can leverage their expertise in this area and whom we work closely with to help us identify work that would be impactful. The second challenge would be data quality and availability.
AI/ML models require large quantities of high-quality data for training and validation. And for supervised models, we need labeled data. Before we greenlight a project, the team spends time doing exploratory analysis on the data to see if there is sufficient high-quality data available. We also work closely with a team of data review experts who provide us with the labeled data that we need.
Finally, and one of the greatest challenges, is resistance to adoption. We try to mitigate that through clear communication and education, demonstrating the tangible benefits of AI/ML adoption, and, for now, focusing on augmentation, not replacement.
Venu: Can you provide a sneak peek into exciting use cases you are working on and how they will benefit the industry?
Sandy: One example is the detection of appropriate concomitant medication-indication pairs, that is, whether a conmed given for an indication is medically appropriate. Another example is the surfacing of similar records to a record of interest according to a user defined criteria. We’ll also be adding an algorithm to rank order records, from riskiest to least risky, of all records flagged by our AI models. The ordered list will be grouped by record, subject, and site.
In addition to data review automation, we are also working on AI augmented data classification and mapping. For AI augmented data classification, our first use case is on building visualizations using a common data model, or a common vocabulary, so that users can build visualizations without having to know the source names which may vary from study to study. We are using NLP to match source names to the common vocabulary. For AI augmented mapping, we are leveraging existing mapping specifications to automatically generate transformations without manual coding.
Venu: Finally, what is your elevator pitch to skeptics of AI/ML application in Clinical Data Analytics or Life Sciences in general?
Sandy: By leveraging the power of artificial intelligence and machine learning, we can unlock invaluable insights from vast patient datasets, enabling early disease detection, precision medicine, and predictive healthcare trends, and improving the efficiency and quality of clinical research.
Listeners interested in seeing eIQ Review in action are invited to watch this recording of the Academy webinar, Introduction to eIQ Review.
Future topics for the Innovation Series podcast include statistical computing with AI/ML, lessons learned from AI implementation, achieving excellence by keeping the “human-in-the-loop”, and more. Subscribe to stay updated on all the latest episodes.
About Sandy Ng
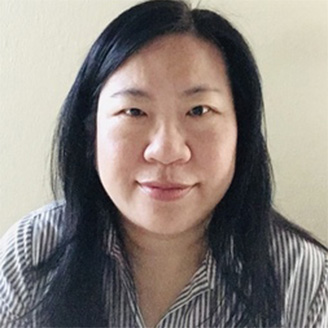
Sandy Ng is the VP of Data Science at eClinical Solutions. Her team collaborates closely with internal and external stakeholders to bring humans-in-the-loop AI/ML solutions to life science problems. She holds a doctorate in engineering and is experienced with building data science products for cardiovascular and neurological applications at startup and midsized medical device companies and at academic medical centers. For the past five years, she has been focused on AI/ML applications to healthcare payer and provider data and clinical trials data.
About Venu Mallarapu
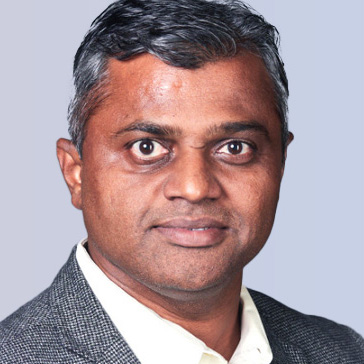
Venu Mallarapu is a digital innovation leader with over two decades of experience in business and IT advisory, strategic consulting, relationship, and delivery management to global life sciences organizations. As eClinical Solutions’ Vice President of Global Strategy and Operations, Venu is responsible for strategic development to meet global demand for the company’s platform and service offerings. He brings his expertise in collaboration and building teams to drive adoption, market expansion and innovation of the elluminate Clinical Data Cloud and eClinical’s Biometrics Services, ensuring current and future clients recognize value as industry needs evolve. Venu is a subject matter expert in Clinical, Regulatory, Quality and Safety & Pharmacovigilance functions, and has delivered Strategy & Transformation Advisory Consulting to top global Pharma, Biotech, Vaccine, and Medical Devices clients. He has spoken at various Life Sciences and Tech industry events and is a recognized thought leader in R&D with published articles.
Author
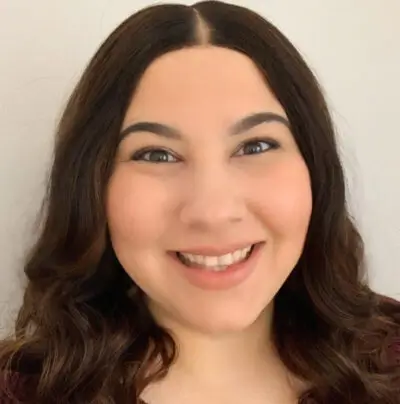
By submitting, you agree to the processing of your personal data by eClinical Solutions as described in our Privacy Policy.